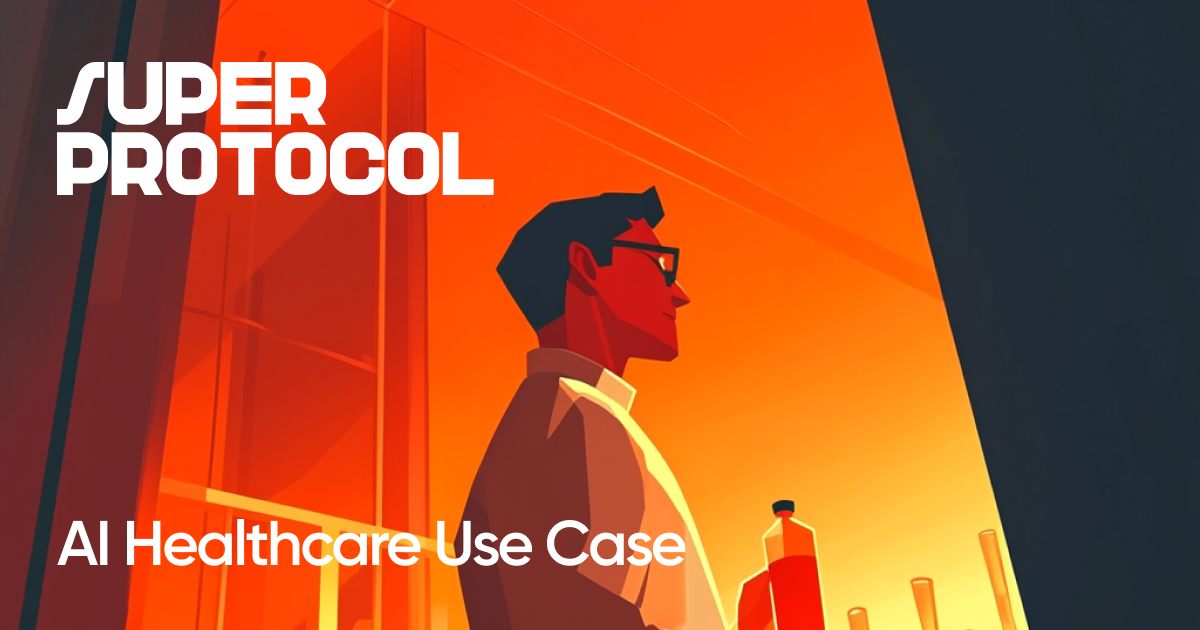
How AI Doctors Collect User Data and How Confidential Computing Mitigates Security Risks
AI-powered healthcare services require vast amounts of sensitive user data to function effectively. Learn how confidential computing can address the inherent security and privacy challenges.
Super Team
Personal AI doctors, such as Ada Health, K Health, Babylon Health, and others, use artificial intelligence to analyze user symptoms, provide preliminary medical assessments, and suggest treatment recommendations. However, to function effectively, these AI models require vast amounts of user health data, posing significant privacy and security risks.
How AI Healthcare Services Gather User Data
These AI healthcare services gather user data in several ways:
- Direct user input – Users manually enter symptoms, age, medical history, and lifestyle habits.
- Medical case histories and diagnoses – AI learns from real patient cases, analyzing how specific symptoms correlate with diagnoses and treatments.
- Integration with medical systems – Some AI doctors connect with electronic health records (EHR/EMR) via API access.
- Analysis of anonymized data – Companies may collect de-identified data to improve model accuracy and reliability.
Once collected, the data is processed and used to train neural networks, which:
- Identify symptom patterns and their correlations with diseases.
- Learn to predict the likelihood of different medical conditions.
- Improve continuously by analyzing millions of patient interactions.
Handling such sensitive data introduces critical risks: data breaches, regulatory non-compliance, and user distrust. People hesitate to share their health data with AI services if they are unsure about data security.
How Confidential Computing Can Solve These Issues
Confidential Computing can drastically enhance the security of AI-driven healthcare by minimizing data exposure and eliminating unauthorized access risks.
1. Encrypted Data Processing
Unlike standard methods where data must be decrypted before analysis, Confidential Computing allows AI models to process data while it remains encrypted. This ensures that even the service providers cannot see or misuse users' health data.
2. Model Training Inside a Secure Environment (TEE)
AI doctors can train their models within isolated computing environments, where:
- Access to data is strictly controlled at the hardware level.
- Even cloud service administrators cannot access raw data.
- Data is automatically deleted after processing, preventing leaks or unauthorized use.
3. Decentralized Data Processing
Instead of sending all user health data to a centralized cloud, AI models can process it locally on the user's device or within encrypted environments on secure servers. This reduces the risk of data exposure and ensures compliance with strict privacy regulations.
4. Access Control via Smart Contracts
Confidential Computing can leverage smart contracts to define exactly which data AI can access and under what conditions. This provides transparency and control for users over their medical information.
Conclusion
By implementing Confidential Computing technology, AI healthcare providers can offer users the benefits of AI-powered medical assistance while maintaining the highest standards of data privacy and security. This approach builds trust with users and ensures compliance with increasingly strict data protection regulations worldwide.